Introduction: The Potential of AI in Bicycle Design
As the cycling industry continues to blend traditional craftsmanship with cutting-edge technology, a new contender is rapidly gaining ground: artificial intelligence. What was once the realm of science fiction is now a real-world game-changer. AI is stepping into the design studio, engineering lab, and manufacturing floor, promising to revolutionize how bicycles are created, tested, and tailored to individual riders.
The cycling world has already embraced various technologies—from carbon fiber frames to electronic shifting and integrated data sensors. But AI introduces a new level of design intelligence, enabling machines to learn from rider data, analyze performance variables, and generate bike geometries that are both optimized and personalized. Instead of relying solely on human intuition or general fitting charts, AI allows for a deep dive into biomechanics, terrain preferences, and riding habits.
This raises a compelling question: are we on the brink of a design evolution where no two bikes are exactly alike, because each is engineered specifically for the rider? In this article, we’ll explore how AI is reshaping customization in cycling, what industry experts are saying, how bike brands are responding, and whether AI-driven design is the future of bike innovation or simply a trend on the horizon.
Customization Through AI: How Machine Learning Could Tailor Bikes to Individual Needs
Customization has always been a prized concept in cycling. Professional riders fine-tune every element of their bikes—from handlebar width to crank length—to maximize performance. For everyday cyclists, a comfortable fit can make or break their riding experience. AI is now positioned to make that level of personalization accessible to all.
Machine learning algorithms thrive on data. By feeding them rider-specific metrics such as height, inseam, riding posture, power output, heart rate, and even terrain preferences, AI systems can generate bespoke bike designs with uncanny accuracy. These systems consider multiple variables simultaneously and can optimize for specific goals—be it speed, endurance, comfort, or handling.
Imagine visiting a bike shop where, instead of choosing from preset frame sizes, you step into a 3D body scanner that captures your anatomy and riding posture. In minutes, the AI recommends an optimal frame geometry, ideal gear ratios, and suggested materials based on how and where you ride.
Companies are also using AI for real-time feedback loops. Bikes equipped with smart sensors transmit performance data back to manufacturers, who analyze it to improve future models. Some software platforms can even simulate the behavior of a bike design under various riding conditions—wind resistance, torque distribution, and stress loads—eliminating the need for multiple prototypes.
This approach not only enhances rider experience but also cuts waste, lowers production costs, and supports faster innovation cycles. It transforms bike design from a one-size-fits-all approach to a continuous learning process, always adapting and improving based on real-world use.
Expert Insights: What Designers and Engineers Think About AI in Bike Manufacturing
To gauge the true impact of AI in bike design, we turn to the people shaping the future: designers, engineers, and researchers at the forefront of cycling innovation.
Dr. Lena Hofmann, Lead Engineer at Velotech Innovations:
“AI gives us a tool we never had before—a way to analyze millions of ride scenarios without ever touching a wind tunnel. It’s like having a lab that runs 24/7, constantly learning and improving the model.”
Jake Nguyen, Industrial Designer at a boutique bike company in Portland:
“Traditionally, we’d rely on experience, customer feedback, and a bit of gut instinct. With AI, we’re building bikes based on predictive modeling, not just historical trends. The level of accuracy in design is incredible, especially when we combine AI outputs with 3D printing for prototyping.”
Priya Mahajan, Materials Scientist and Consultant:
“AI helps us not just in geometry but in materials optimization. It can suggest layered carbon patterns or aluminum blends that improve ride feel while reducing weight—things that would take months of testing before.”
Lucas Romero, Head of Custom Builds at a major brand:
“We’ve started integrating AI to streamline our custom-order process. It helps shorten lead times, improves fit rates, and enhances customer satisfaction. The next step is true mass customization, and AI is the key to unlocking it.”
While excitement is high, experts also note potential limitations. Data quality is paramount—flawed input leads to flawed output. And there’s an ongoing need for human oversight. After all, bikes are not just machines; they’re extensions of our bodies and expressions of identity. AI enhances the process, but doesn’t replace the craft.
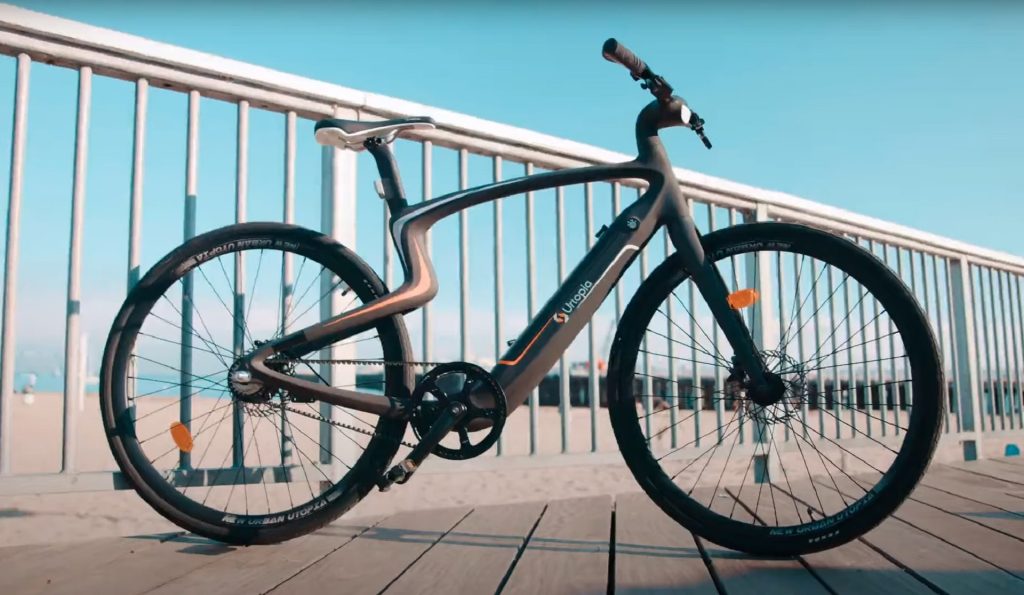
Industry Response: Are Bike Brands Ready to Adopt AI for Mass Customization?
Bike brands are notoriously slow to adopt sweeping technological change, especially when it challenges traditional methods. However, the tide may be turning. Several major manufacturers and startups are already experimenting with AI-driven tools, both in-house and through collaborations with tech firms.
Specialized, for instance, has partnered with AI analytics companies to refine their Body Geometry Fit system, moving beyond static measurements to dynamic data-based suggestions. Their high-end bikes now come with embedded sensors that collect real-time metrics, which can then inform future iterations of design.
Canyon and Trek are exploring AI to optimize their supply chains and better predict demand trends, which in turn influences product development. They’re also investing in AI-assisted design software to shorten their R&D cycles.
Factor Bikes, known for performance-oriented builds, is piloting a rider DNA program where biometric data feeds directly into frame design. Early results suggest a measurable improvement in rider efficiency and reduced fatigue.
Meanwhile, smaller custom brands are leading the charge. Companies like Alchemy Bicycles, No. 22, and Moots are already offering AI-informed custom builds, combining deep-fit analysis with generative design tools.
Despite enthusiasm, widespread adoption still faces hurdles. Cost is a major factor—AI infrastructure and specialized staff require significant investment. There are also questions around data privacy, intellectual property, and scalability.
However, as AI tools become more affordable and user-friendly, and as customer demand for personalized products increases, industry adoption is likely to accelerate.
Conclusion: Will AI Become a Staple in Cycling Design?
The rise of AI in bicycle design is not just an incremental improvement; it’s a paradigm shift. We’re moving from reactive to predictive, from standardization to personalization, and from trial-and-error to data-driven optimization. AI enables designs that adapt to the rider, not the other way around.
Yet, the future of AI in cycling is not solely about technology—it’s about how the industry balances innovation with identity. Cyclists value performance, but they also cherish craftsmanship, legacy, and style. The success of AI will depend on how well it can integrate into this culture, not override it.
Looking forward, AI is poised to become a staple—not just for elite athletes or custom builds, but for everyday riders seeking a better fit, a smoother ride, or a deeper connection with their bikes. Whether used in design, materials selection, real-time feedback, or production workflows, AI offers a toolkit for the next generation of cycling.
In a world where personalization is increasingly the norm, AI may well be the key that unlocks the perfect ride for every rider. And that’s a revolution worth riding for.
Discussion about this post