In the dynamic realm of cycling innovation, a new force is beginning to redefine how bicycles are imagined, developed, and built: artificial intelligence. Once limited to data analysis and automation, AI has now ventured into the creative and engineering domains of bicycle design. It is reshaping traditional R&D workflows, accelerating prototyping, optimizing performance metrics, and even predicting market trends with uncanny accuracy. As sustainability, efficiency, and personalization become central themes in cycling’s evolution, the role of AI in design and manufacturing is no longer experimental—it is essential. This article explores how AI is carving a new frontier in bicycle design and what that means for the future of cycling from the workshop to the saddle.
From Data Crunching to Creative Collaboration
Artificial intelligence in bicycle design begins with data—lots of it. Every pedal stroke, wind tunnel session, frame vibration, and crash test generates troves of data that once took teams of engineers months to process. Now, AI models can analyze that information in real-time, identifying patterns and potential design improvements with speed and precision. More impressively, AI isn’t just a tool for analysis—it’s becoming a creative collaborator. Generative design algorithms use machine learning to explore thousands of potential frame geometries or component shapes based on parameters like weight, stiffness, aerodynamics, and rider type. Designers input constraints such as material limits or cost ceilings, and the AI suggests forms that a human might never have considered. It’s not just about streamlining the process—it’s about breaking the boundaries of what’s possible.
Revolutionizing Frame Geometry and Aerodynamics
Frame geometry has always been the heart of bicycle design, influencing comfort, control, and power transfer. Traditional methods relied on empirical testing, rider feedback, and iterative prototyping. Today, AI can simulate rider movement, posture, and forces in virtual environments to predict how a frame geometry will perform before a single tube is cut. By incorporating feedback from real-world sensors—such as power meters, cadence sensors, and gyroscopic data—AI models can fine-tune geometry to specific use cases like endurance riding, criteriums, or gravel adventures. Additionally, AI-driven computational fluid dynamics (CFD) simulations allow designers to test how different frame shapes interact with airflow, helping them shave off critical seconds in time trials. Specialized’s use of AI in aerodynamic optimization led to the creation of their S-Works bikes with noticeably less drag and improved stiffness-to-weight ratios, showcasing the tangible performance benefits of AI-enhanced design.
Material Science Meets Machine Learning
The bicycle industry has undergone several revolutions in materials, from steel and aluminum to carbon fiber and now, hybrid composites. AI plays a pivotal role in selecting and optimizing these materials. Machine learning models can predict how different fiber weaves, resin compositions, and layup patterns will respond under stress—something that once required extensive physical testing. Manufacturers like Trek and Canyon are now using AI to model material behavior in microseconds, reducing the time and cost of development. Even more intriguing is the integration of AI in the 3D printing of bespoke components. AI helps calculate optimal infill patterns, weight distributions, and support structures, resulting in stronger and lighter parts with less waste. This combination of additive manufacturing and intelligent algorithms is opening the door to ultra-customized frames, stems, and saddles tailored not just to a rider’s size but to their unique biomechanics.
AI-Driven Personalization and Fit
No two riders are alike. From femur length and riding discipline to flexibility and injury history, each cyclist has unique needs that traditional bike sizing charts often overlook. AI is bridging this gap with hyper-personalized solutions. Platforms like Retül and GURU have incorporated AI to analyze rider motion capture data and recommend precise frame sizing, saddle position, and component selection. Going a step further, some direct-to-consumer bike brands now offer AI-based configurators where riders input data (or upload a ride file), and the system outputs a recommended geometry, component setup, and even wheel size. Imagine a future where a bike is not just made for a category of rider, but for you—your body, your riding style, and your aspirations—created through AI-powered design pipelines.
Enhancing Manufacturing Efficiency and Sustainability
Beyond design, AI is revolutionizing bicycle manufacturing through smart factories and predictive maintenance. Robotic arms guided by AI can precisely cut carbon fiber sheets, apply resin, or weld aluminum frames with minimal human oversight. Predictive maintenance algorithms help machinery stay in peak condition, reducing downtime and energy waste. AI also enables demand forecasting, helping brands avoid overproduction and reduce excess inventory—a major sustainability concern in the cycling industry. Some companies are even using AI to track environmental impact across the supply chain, optimizing transport routes, sourcing decisions, and packaging based on carbon footprint predictions. The result is a more agile, eco-conscious manufacturing process that aligns with the growing consumer demand for responsible production.
Rider Behavior and Market Insights
AI’s capabilities extend far beyond design and production—it also helps brands understand riders better than ever. By analyzing data from ride-tracking apps like Strava, Garmin Connect, or Zwift, companies can identify shifts in terrain preference, average ride duration, and performance goals. AI can then feed this information back into product design, marketing strategies, and feature development. For example, the recent boom in gravel biking was partly predicted through AI trend analysis of user data, leading to a surge in adventure-oriented models. AI tools can also personalize marketing content, recommending bikes or gear based on a rider’s behavior history, previous purchases, or fitness level. This feedback loop between rider activity and product development ensures that innovation remains in sync with real-world needs.
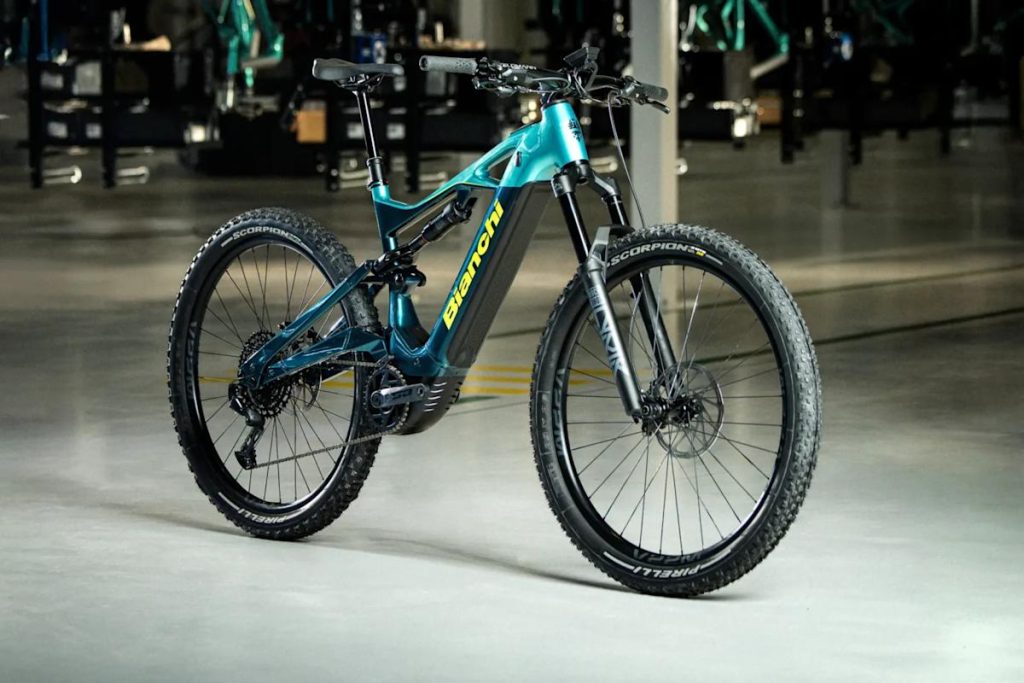
Safety Features and Real-Time Adjustments
As AI moves into the active components of bikes, new frontiers are opening for safety and ride quality. Smart suspension systems, powered by AI and onboard sensors, can adjust compression and rebound in real-time based on terrain, speed, and rider weight. Canyon’s KIS (Keep It Stable) system uses a combination of machine learning and mechanics to assist with high-speed stability. In urban settings, AI-enhanced e-bikes are beginning to feature collision detection, predictive braking, and lane-change warnings. These capabilities, borrowed from automotive autonomous systems, could significantly reduce accidents and make cycling safer for commuters and recreational riders alike. Eventually, AI could help govern traffic flow for cyclists in smart cities, where bike infrastructure communicates with riders to avoid congestion or alert them of hazards.
Challenges and Ethical Considerations
Despite its promise, the integration of AI in bicycle design raises important challenges. Data privacy is a key concern, especially when rider biometric or location data is collected to feed AI systems. Manufacturers must prioritize transparency and security to build trust. There’s also the question of accessibility—will AI-designed bikes remain a luxury, or can the cost savings from efficient design and production be passed on to consumers? Additionally, reliance on AI could potentially stifle human creativity if not balanced properly. Designers must continue to be empowered as artists and innovators, with AI as a partner rather than a replacement. Finally, environmental responsibility must remain central, especially when adopting energy-intensive AI computing processes.
Looking to the Future
The future of AI in bicycle design is as boundless as the imagination itself. As neural networks become more sophisticated and quantum computing enters the scene, we may soon see AI-generated bikes that adapt dynamically to the rider’s effort in real-time, or virtual twin technology that allows engineers to test and iterate entire production lines in a digital realm before manufacturing begins. We could have bikes that diagnose mechanical issues autonomously, components that optimize themselves based on weather and altitude, or even blockchain-backed design systems that track a product’s entire life cycle. One day, a new rider might scan their body, upload riding data, and receive a tailor-made frame shipped in days—sustainable, ergonomic, and inspired by millions of data points synthesized through a neural net. The collaboration between AI and cycling is still young, but the direction is clear: more personalized, more efficient, and more innovative than ever before.
Cycling has always been a blend of simplicity and sophistication, from the humble penny-farthing to the carbon-fiber marvels of today. As AI enters the design studio and the factory floor, it doesn’t erase the soul of cycling—it enhances it. By empowering designers with deeper insights and giving riders more personalized tools, artificial intelligence is setting the stage for a new golden age of bicycle innovation. For the cycling community, it’s an exciting ride into uncharted terrain—with AI along for the journey, one pedal stroke at a time.
Discussion about this post