In the evolving world of cycling, where innovation often races ahead of tradition, a new technological force is beginning to reshape how riders and professionals approach one of the most essential—but often overlooked—aspects of cycling: maintenance. Artificial Intelligence (AI), once confined to the realms of sci-fi and data labs, is now rolling into the garages and bike shops of everyday cyclists, promising smarter, faster, and more accurate diagnostics than ever before. But does this integration signal a revolutionary shift or is it merely a convenient upgrade? This article dives deep into the rise of AI-powered bike maintenance tools, their real-world applications, and how they’re redefining the cycling experience from spokes to saddle.
The Evolution of Bike Maintenance: From Manual Wrenches to Smart Warnings
Traditionally, maintaining a bike involved a combination of keen observation, experience, and a well-worn toolkit. Cyclists would rely on the feel of their brakes, the sound of their chain, or the visible wear on their tires to gauge when something needed attention. While that hands-on approach remains valuable, it has its limits—especially as modern bikes become increasingly complex, with advanced drivetrains, integrated electronics, and electric motors. Enter AI, which brings the ability to monitor, detect, and even predict maintenance needs using data collected from sensors, connected devices, and machine learning algorithms.
AI-powered bike maintenance tools function much like a digital mechanic. They gather data from various sources—such as GPS rides, cadence sensors, torque measurements, and even weather conditions—and use algorithms to interpret when components are under stress, nearing failure, or operating inefficiently. Some tools can even issue alerts via smartphone apps or onboard displays, suggesting repairs before issues worsen or lead to breakdowns.
What Are AI-Driven Maintenance Tools?
AI-driven maintenance tools are systems that use artificial intelligence and machine learning to diagnose issues, schedule repairs, and optimize the performance of a bicycle. These tools vary widely in function and form, including:
- Smartphone apps that analyze ride data and provide maintenance recommendations.
- Built-in diagnostic systems in electric bikes that monitor motor and battery health.
- Sensor-equipped components like chains, derailleurs, and brake pads that track wear and alert users.
- Cloud-based platforms for fleet maintenance (e.g., bike-share or delivery services) that aggregate data from thousands of bikes and use AI to optimize service schedules.
- Self-learning systems that adapt recommendations based on rider behavior and environmental conditions.
These tools don’t just make maintenance smarter—they also make it more proactive. Instead of waiting for a chain to break or a derailleur to misalign, AI can detect anomalies early and suggest preemptive fixes.
How AI Improves Diagnostic Accuracy
The true power of AI lies in its pattern recognition. While a human mechanic might spot signs of wear based on a brief inspection, AI tools can process thousands of data points in real time, spotting trends invisible to the naked eye. For example:
- A slight decrease in pedaling efficiency might signal drivetrain misalignment.
- Inconsistent torque readings could indicate loose crank arms.
- Abnormal vibration patterns can flag issues in tire pressure or wheel trueness.
- Battery performance degradation in e-bikes can be predicted with high accuracy, enabling timely replacements.
Over time, these tools “learn” from the rider’s habits and environment. An AI tool that monitors a commuter in rainy London will offer different maintenance schedules than one used by a mountain biker in dry Arizona.
User Adoption: Are Riders Ready for AI?
While the technology is promising, the human side of the equation matters just as much. Are cyclists actually using these tools—and do they trust them?
Early adopters, especially in the e-bike and performance cycling communities, are embracing AI with enthusiasm. Apps like Shimano’s E-Tube, Bosch’s eBike Flow, and startups like Bikefair and See.Sense are seeing increasing engagement. These tools offer a mixture of diagnostics, customization, and repair scheduling that many riders now find indispensable.
Recreational cyclists and casual commuters, however, remain somewhat hesitant. Concerns range from data privacy to perceived complexity and cost. Yet as interfaces become more intuitive and integration with existing platforms (like Strava or Apple Health) improves, adoption is likely to grow—especially if AI proves to reduce repair costs and downtime.
Real-World Use Cases and Success Stories
1. Fleet Optimization in Bike-Sharing: Companies like Lime and Bird have started integrating AI diagnostics in their fleets. These systems monitor usage, wear patterns, and weather-related degradation, triggering maintenance dispatches before bikes fail in the field. As a result, downtime is reduced, user satisfaction improves, and operational costs decrease.
2. Pro Racing Teams: Elite cycling teams have begun using AI tools to manage the health of high-performance bikes. Systems track rider power output in relation to component stress, allowing technicians to swap parts before they compromise a race. This proactive strategy helps prevent in-race mechanical issues that could cost podium spots.
3. Urban Commuters: AI-powered maintenance alerts on commuter bikes help riders avoid surprise breakdowns. Some e-bike models automatically alert the nearest service center when problems arise, and in some cities, mobile bike techs are dispatched accordingly.
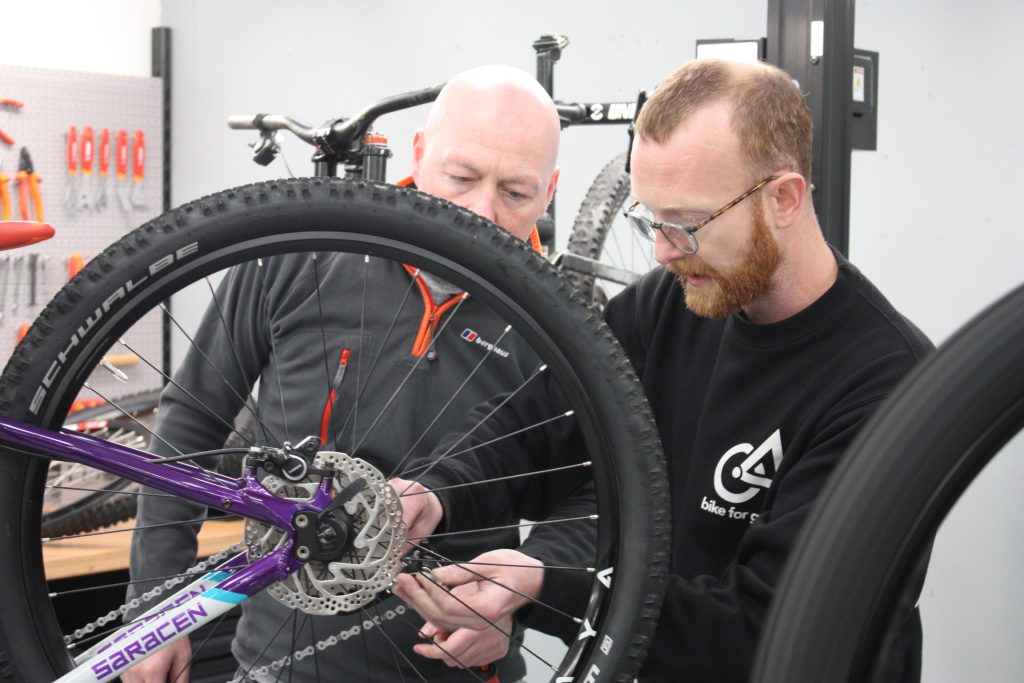
AI vs Human Mechanics: Competition or Collaboration?
Does AI threaten to replace the role of traditional bike mechanics? Not likely. Instead, AI is proving to be a complementary force—helping mechanics become more efficient and accurate. By flagging potential issues in advance, AI allows service professionals to prioritize urgent tasks and ensure that maintenance is precise and data-backed.
Moreover, in workshops using AI-driven service platforms, customer trust is improving. Clients appreciate seeing diagnostic readouts and predictive timelines—it transforms vague advice like “your brakes might need replacing soon” into “your brake pads have 15% wear left and should be replaced within 200km.”
Challenges and Limitations
Despite the promise, several hurdles stand between AI tools and mainstream adoption:
1. Hardware Dependency: Many AI tools require compatible sensors or components, often adding cost and complexity.
2. Learning Curve: Users unfamiliar with bike mechanics may find the data overwhelming or confusing. Simpler, more visual interfaces are needed to translate analytics into action.
3. Privacy and Data Ownership: As with any connected system, users are wary of where their data goes and how it’s used. Transparency and opt-in models are crucial for trust.
4. Standardization Issues: The cycling industry still lacks universal standards for data protocols, making it difficult to create one-size-fits-all tools that work across brands.
5. False Positives/Negatives: AI isn’t perfect. Incorrect alerts can annoy users or lead to unnecessary service visits, while missed issues could damage confidence in the system.
Expert Perspectives: Mechanics, Engineers, and Cyclists Speak
Carlos Mendes, a veteran bike shop owner in Lisbon, says: “AI helps me diagnose faster, especially when a customer doesn’t know what’s wrong. The tools give me direction—I still do the fine-tuning.”
Elena Rojas, an e-bike commuter in Mexico City, notes: “Before AI tools, I often ignored maintenance until something failed. Now my app warns me when to lube the chain or check the brakes—it’s like having a bike coach.”
Arvind Kapur, an AI systems engineer in Amsterdam, explains: “The future is personalized maintenance. Your bike will know you, your routes, your habits. It’ll adjust settings and service intervals just like a smart car.”
What the Future Holds: Predictive, Autonomous, and Cloud-Connected
Looking ahead, the next wave of AI innovation will likely involve deeper integration with IoT (Internet of Things), enabling real-time cloud-based diagnostics, AI-driven service scheduling, and even automated part ordering. Some startups are experimenting with self-healing bikes, where micro-adjustments to chain tension, tire pressure, or brake alignment happen automatically through AI-controlled mechanisms.
Subscription-based models may emerge where cyclists pay a monthly fee for full AI-powered maintenance coverage, including remote diagnostics, at-home tune-ups, and 24/7 support.
Eventually, AI may enable fully autonomous diagnostics kiosks at bike-sharing stations or urban hubs, allowing users to scan their bikes and receive instant health reports before starting their commute.
Conclusion: Game Changer or Glorified Gadget?
The integration of AI into bike maintenance tools is more than just a tech trend—it’s a meaningful shift toward data-driven, efficient, and user-friendly cycling care. While it won’t replace traditional tools or the human touch, it’s already proving invaluable for certain user groups and applications. As hardware becomes more affordable, interfaces more intuitive, and trust in AI grows, we can expect adoption to rise across the board.
For city cyclists looking to avoid roadside mishaps, for mechanics aiming to sharpen their diagnostic accuracy, and for fleet operators seeking scalable solutions, AI may very well be the game changer the cycling world didn’t know it needed—but now won’t want to live without.
Discussion about this post